Courses in Summer Term 2025
We do not offer any courses in Summer Term 2025. We still offer, e.g., student theses and research projects, as well as exams.
You may also refer to the overview in C@MPUS.
In case you are interested in student theses please consult the information here.
Courses in Winter Term 2024/2025
The following courses will be offered in winter term 2024/2025. Detailed information on the courses can be found via the provided C@mpus and Ilias links.
Teaching Statement
Teaching at SQA deals with engineering software systems in a way that they fulfil their functional, but in particular their non- or extra-functional properties. In all kinds of courses we try to connect our topics with current trends and practices in industry. In particular, in most courses employees of our industry partners give lectures, participate in theses or projects, contribute to seminars, etc.
Previous Semesters
The following courses were offered in winter term 2024/2025.
Detailed information on the courses can be found via the provided C@mpus and Ilias links.
- Programmierung und Softwareentwicklung (C@mpus)(Ilias)
- Vortragsübungen Programmierung und Softwareentwicklung (C@mpus)
- Advanced Software Engineering (PO 2017: Software Engineering) (C@mpus)(Ilias)
- Software-Architektur (C@mpus)(Ilias)
- Modeling and Auto-Layouting of Ontological Software Architecture Views (C@mpus)
The following courses were offered in winter term 2023/2024.
Detailed information on the courses can be found via the provided C@mpus and Ilias links.
The following courses were offered in winter term 2022/2023.
Detailed information on the courses can be found via the provided C@mpus and Ilias links.
As a consequence of the recent developments concerning the Corona virus, all our courses will (initially) be held as online courses. Please follow the instructions in the respective ILIAS courses and contact the organizers if needed. Further general information can be found on the university web site: https://www.uni-stuttgart.de/universitaet/aktuelles/meldungen/corona/
The following courses were offered in summer term 2022.
Detailed information on the courses can be found via the provided C@mpus and Ilias links.
As a consequence of the recent developments concerning the Corona virus, all our courses will (partially) be held as online courses. Please follow the instructions in the respective ILIAS courses and contact the organizers if needed. Further general information can be found on the university web site: https://www.uni-stuttgart.de/universitaet/aktuelles/meldungen/corona/
The following courses were offered in winter term 2021/2022.
Detailed information on the courses can be found via the provided C@mpus and Ilias links. In case you are interested in student theses please consult the information here.
As a consequence of the recent developments concerning the Corona virus, all our courses will (initially) be held as online courses. Please follow the instructions in the respective ILIAS courses and contact the organizers if needed. Further general information can be found on the university web site: https://www.uni-stuttgart.de/universitaet/aktuelles/meldungen/corona/
The following courses were offered in summer term 2021.
Detailed information on the courses can be found via the provided C@mpus and Ilias links. In case you are interested in student theses please consult the information here.
As a consequence of the recent developments concerning the Corona virus, all our courses will (initially) be held as online courses. Please follow the instructions in the respective ILIAS courses and contact the organizers if needed. Further general information can be found on the university web site: https://www.uni-stuttgart.de/universitaet/aktuelles/meldungen/corona/
The following courses were offered in winter term 2020/2021.
Detailed information on the courses can be found via the provided C@mpus and Ilias links. In case you are interested in student theses please consult the information here.
- Requirements Engineering und Software-Architektur (C@mpus)(Ilias)
- Software Engineering (C@mpus)(Ilias)
- Programmierung und Softwareentwicklung (C@mpus)(Ilias)
- Vortragsübungen Programmierung und Softwareentwicklung (C@mpus)
- Seminar Selected Topics in Software Engineering: Software Quality Verification and Forecasting (C@mpus)(Ilias)
As a consequence of the recent developments concerning the Corona virus, all our courses will (initially) be held as online courses. Please follow the instructions in the respective ILIAS courses and contact the organizers if needed. Further general information can be found on the university web site: https://www.uni-stuttgart.de/universitaet/aktuelles/meldungen/corona/
The following courses were offered in summer term 2020.
Detailed information on the courses can be found via the provided C@mpus and Ilias links. In case you are interested in student theses please consult the information here.
The following courses were offered in winter term 2019/2020.
Detailed information on the courses can be found via the provided C@mpus and Ilias links.
- Requirements Engineering und Software-Architektur (C@mpus)(Ilias)
- Software Engineering (C@mpus)(Ilias)
- Programmierung und Softwareentwicklung (C@mpus)(Ilias)
- Vortragsübungen Programmierung und Softwareentwicklung (C@mpus)
- Seminar Selected Topics in Software Engineering: Software Quality Verification and Forecasting (C@mpus)(Ilias)
The following courses were offered in summer term 2019.
Detailed information on the courses can be found via the provided C@mpus and Ilias links.
The following courses were offered in winter term 2018/2019.
Detailed information on the courses can be found via the provided C@mpus and Ilias links. In case you are interested in student theses please consult the information here.
Partners
Visual Paradigm provides University of Stuttgart with UML and BPMN tools under the Academic Partner Program.
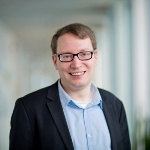
Steffen Becker
Prof. Dr.-Ing.Head of the Software Quality and Architecture Group
[Image: U. Regenscheit / University of Stuttgart]